Large Language models (LLMs) have changed the Search landscape. They have created a new direction in user behaviour, offering internet users a previously unseen way to obtain information and receive answers to questions in a much more natural and ‘human’ manner.
Large Language Models (LLMs) have changed the Search landscape. They have reshaped user experience in the most profound ways. By providing a more conversational, human-like method of obtaining information, LLMs (Chat GPT, Claude, DeepSeek etc), offer users a seamless and natural way to get answers to their questions; something traditional search engines like Google, have never fully achieved.
These models are not just another iteration of traditional search engines—they are fundamentally different in how they source, process, and generate information.
In this article, we’ll explore how LLMs source information and how their approach contrasts with traditional search engines like Google.
What Are LLMs and How Do They Work?
LLMs are advanced artificial intelligence systems trained on vast amounts of text data. They function by predicting and generating human-like text based on the input they receive. Rather than simply retrieving a list of links or web pages like traditional search engines, LLMs create contextualised responses tailored to the user’s query. They do this by processing information from a range of sources they’ve been trained on, which could include books, websites, academic papers, and other publicly available text-based content.
The main strength of LLMs lies in their ability to understand natural language and context, enabling them to answer questions, offer explanations, and even engage in multi-turn conversations. They generate answers by synthesising information learned during training, rather than pulling directly from specific documents or web pages. This allows them to respond quickly, offering answers that can seem like they are drawn from a single, cohesive source.
How LLMs Differ from Traditional Search Engines
Traditional search engines like Google, on the other hand, work on a fundamentally different principle.
When you type a query into Google, the engine doesn’t directly “understand” your question in the way LLMs do. Instead, it uses complex algorithms to crawl and index billions of web pages and rank them according to relevance. These rankings are based on various factors, including keywords, backlinks, user experience, and content quality. Google then serves you a list of links to web pages it deems most relevant to your search query.
This difference in approach is key. Traditional search engines rely heavily on a link-based model. Google serves up a list of resources, expecting the user to sift through them to find the answer to their query. The searcher is often left to parse through several web pages to find the right information, sometimes not getting the exact answer they are looking for immediately.
In contrast, LLMs work by generating direct answers. Instead of listing resources or requiring users to click through multiple results, LLMs offer a synthesised, concise response based on the information they’ve learned. This is a much more conversational, seamless experience for users, particularly when the query is straightforward or specific.
How LLMs Source Their Information
LLMs derive their information from large datasets gathered during their training phase. These datasets can include books, articles, research papers, websites, and other publicly available content. However, it’s crucial to note that LLMs don’t “search” the web in real-time for information—unless configured with browsing capabilities. Traditional LLMs rely on their pre-existing training, meaning the information they provide may not always be current or accurate, especially when it comes to rapidly changing topics like news or recent events.
For example, when you ask an LLM like ChatGPT a question about a historical event, it will synthesise or consolidate an answer based on the information it was trained on. However, if you ask about a recent event, the model may not provide the most up-to-date answer since it doesn’t pull real-time data from the web—unless the LLM is using real-time browsing capabilities.
Real-Time Search in LLMs: A Growing Capability
While traditional LLMs like ChatGPT don’t pull live data from the internet during interactions, real-time search capabilities are starting to be incorporated into some models and platforms.
For instance, certain applications of LLMs—such as ChatGPT with browsing enabled or other specialised AI systems—allow for real-time searches across the internet. This means that users can ask LLMs to retrieve up-to-date information, news, reviews or even access live data from websites.
By integrating real-time browsing functionality, these models are able to overcome one of the biggest limitations of older LLM systems, where responses are static and outdated by nature. With browsing enabled, LLMs retrieve information directly from the web, often citing the sources from which they pull the data, much like traditional search engines provide links to results.
For example, when browsing is enabled, if you ask an LLM about a recent news event, it will search the web for the most relevant and up-to-date articles or information and provide a direct answer, along with links to the sources it referenced.
This capability bridges the gap between traditional search engines and LLMs, allowing AI-driven models to offer current, source-backed answers.
The Strengths and Limitations of LLMs
One of the strengths of LLMs is their ability to synthesise information from various sources and offer more nuanced, context-rich answers. This makes them ideal for queries that require explanation or a complex consolidation of multiple pieces of information (from multiple sources). For instance, if you asked an LLM about the concept of “quantum mechanics,” it would generate a response that includes the core principles, definitions, and related concepts, all from a general understanding built from diverse resources.
However, this approach also has its limitations. Since LLMs don’t actively browse the web or access real-time data (unless specifically enabled), their knowledge can become outdated. They can also generate inaccurate or biased responses based on their training data, which can sometimes lead to misinformation or “hallucinations” (false information presented as fact).
LLMs vs. Traditional Search Engines: A Comparison
Aspect | Traditional Search Engines | LLMs |
Approach | Returns a list of relevant web pages based on ranking algorithms | Generates synthesised answers directly from training data |
Data Source | Web crawling and real-time indexing of web pages | Pre-trained on large datasets but does not access real-time data unless browsing is enabled |
Real-Time Search | Can retrieve real-time, up-to-date results | Can integrate real-time search in certain applications |
User Experience | Requires user to sift through results to find answers | Provides direct, contextually relevant answers instantly, with cited sources if browsing is enabled |
Strengths | Up-to-date results, diverse perspectives, access to a wide range of sources | Immediate, conversational answers with contextual understanding |
Limitations | Can result in information overload, may require multiple clicks | Can be outdated, may provide inaccurate or biased responses unless browsing is enabled |
Example | Searching “Where is the best fish restaurant in Newcastle?” gives a list of review articles, maps, and opinions across multiple sites like Yelp, TripAdvisor, and local food blogs | Asking “Where is the best fish restaurant in Newcastle?” provides a direct answer, often consolidating multiple perspectives and sources (review sites, Google reviews, forums) into a single, generalised response. |
Conclusion: The future of LLMs’ takeover of traditional search.
The rise of LLMs marks a significant shift in how we search for and consume information. While traditional search engines like Google excel at providing a list of resources and up-to-date information, LLMs offer a more direct, conversational approach to answering questions.
By understanding the underlying differences in how these systems source and generate information, businesses can better navigate the evolving landscape of search and adjust their digital strategies to optimise for AI-driven search experiences.
As real-time search becomes more integrated into LLMs, the gap between AI-driven search and traditional search engines will continue to narrow, offering exciting new opportunities for both users and marketers.
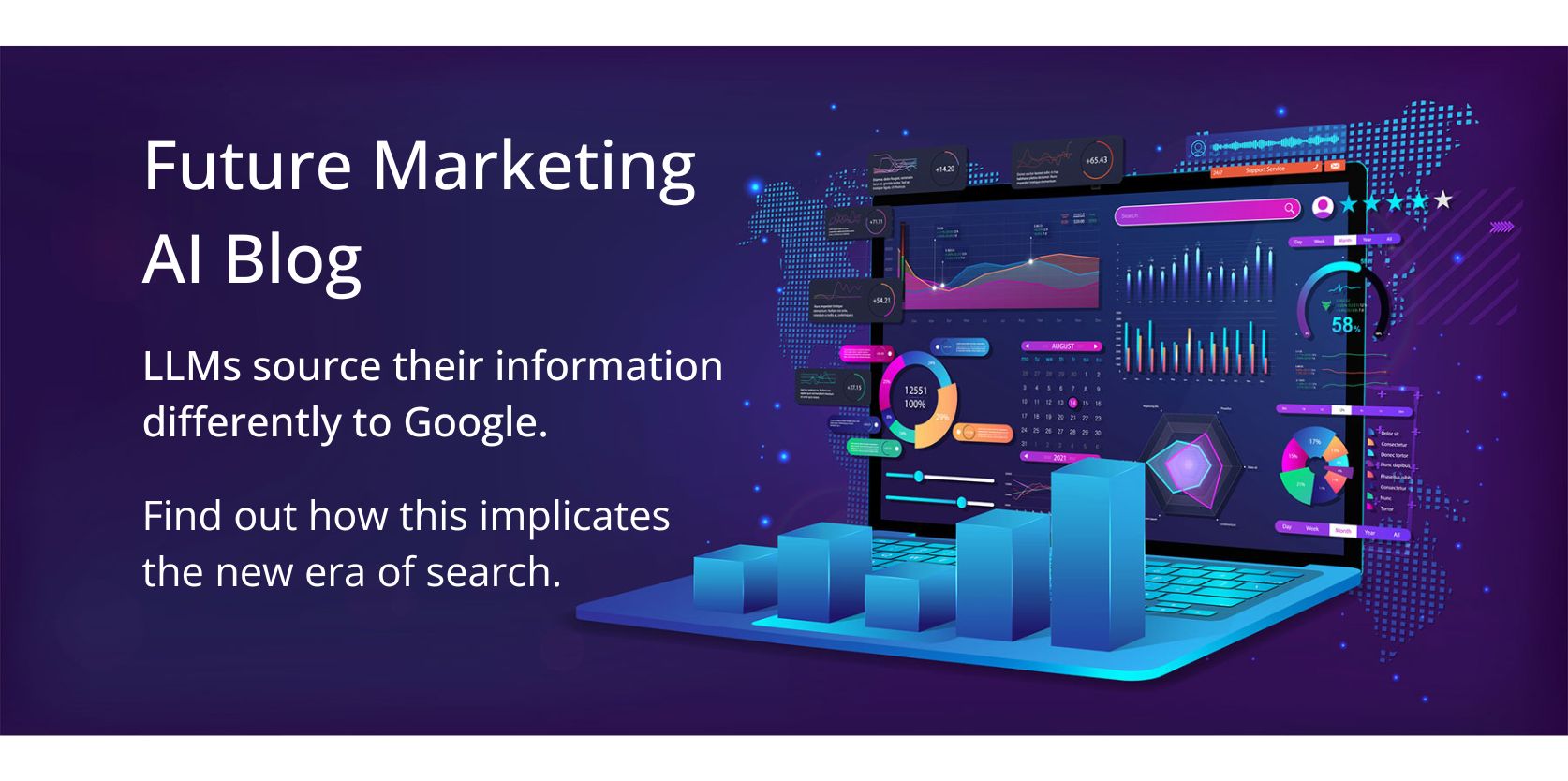